Comprehensive guide to data analytics for marketing professionals
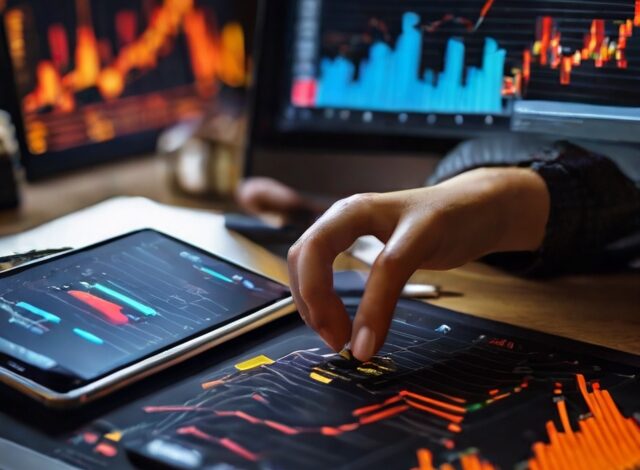
Introduction
In the constantly evolving landscape of marketing, the role of data analytics has become more crucial than ever. As we navigate through a digital era where consumer behaviors, market trends, and competitive landscapes shift rapidly, the ability of marketing professionals to make informed, data-driven decisions is not just a competitive advantage but a necessity.
The Importance of Data Analytics in Marketing
Data analytics in marketing goes beyond the mere collection of data; it involves the systematic analysis of data sets to identify patterns, understand consumer behaviors, and predict future trends. In today’s world, where customers are bombarded with information and choices, understanding what your audience wants, how they behave, and what influences their purchasing decisions can significantly impact the effectiveness of your marketing strategies.
The essence of data analytics lies in its ability to transform raw data into actionable insights. For instance, by analyzing website traffic data, a marketer can understand which pages engage customers the most, or by evaluating social media interactions, they can determine the types of content that resonate with their audience. This level of insight is invaluable in crafting targeted marketing campaigns that not only reach the right audience but also deliver the right message at the right time.
Transforming Marketing Strategies with Data Analytics
Data analytics has revolutionized marketing strategies in several ways. Firstly, it allows for more personalized marketing. By understanding individual customer preferences and behaviors, companies can tailor their marketing messages to resonate more deeply with their audience. For example, e-commerce sites use customers’ browsing and purchase history to recommend products, significantly enhancing the shopping experience and increasing sales.
Secondly, data analytics enables more efficient allocation of marketing resources. By understanding which channels and campaigns are most effective, marketers can optimize their budgets, focusing more on high-performing strategies and less on those with lower returns. This efficiency not only saves money but also maximizes the impact of marketing efforts.
Lastly, data analytics empowers real-time decision making. In the fast-paced digital world, the ability to quickly adapt to changing trends or customer feedback can be the difference between a successful campaign and a missed opportunity. Real-time analytics provide insights that allow marketers to tweak campaigns on the fly, ensuring they remain relevant and effective.
As we delve deeper into this comprehensive guide, we will explore the various facets of data analytics in marketing, from the types of analytics and data collection methods to the tools, technologies, and skills required to leverage this powerful discipline effectively. For marketing professionals keen to stay ahead in their field, understanding and applying data analytics is no longer optional; it’s a critical component of success in the digital age.
Section 1: Understanding Data Analytics in Marketing
Definition and Scope of Data Analytics in Marketing
Data analytics in marketing refers to the process of examining data sets to draw conclusions about the information they contain. This involves applying algorithms and statistical analysis to data to uncover patterns and trends that can inform marketing decisions. In the context of marketing, this data is often related to consumer behavior, market trends, campaign performance, and more.
The scope of data analytics in marketing is vast. It covers everything from basic market research to advanced predictive modeling. It’s about understanding not just who your customers are, but also how they behave, what they need, and how they interact with your brand across various channels. This understanding allows marketers to create more effective campaigns, predict market trends, personalize customer experiences, and measure the success of their efforts with greater precision.
The Evolution of Data Analytics in Marketing
The evolution of data analytics in marketing has been rapid and transformative. In the early days, marketing decisions were largely based on assumptions and limited data sets. Traditional methods like surveys and focus groups provided some insights, but they were often time-consuming and not always representative of the broader market.
With the advent of digital technology and the explosion of data availability, the landscape changed dramatically. Marketers now have access to vast amounts of data, from online consumer interactions to detailed demographic information. Tools and technologies have evolved to not only collect and store this data but also to analyze and interpret it in meaningful ways.
The rise of big data, AI, and machine learning has further advanced the field, enabling more sophisticated analysis and predictive capabilities. Marketers can now anticipate customer needs, forecast market changes, and react in real-time to consumer behavior, setting a new standard for marketing effectiveness.
Key Differences Between Traditional Marketing and Data-Driven Marketing
The shift from traditional marketing to data-driven marketing marks a significant change in how companies approach their marketing strategies. Traditional marketing often relied on broad, generalized strategies aimed at wide segments of the population. It was a one-size-fits-all approach, where the same message was broadcast to all consumers, regardless of their specific interests or behaviors.
Data-driven marketing, on the other hand, is highly targeted and personalized. It uses data analytics to understand individual consumer preferences and tailor messages specifically to them. This approach not only increases the likelihood of engagement but also enhances the customer experience by providing relevant and timely content.
Moreover, traditional marketing often struggled with measuring effectiveness. It was challenging to link a specific marketing effort directly to sales or engagement. Data-driven marketing, with its focus on analytics, allows for precise measurement and analysis of campaign performance. Marketers can track a range of metrics, from click-through rates to conversion rates, giving them a clear understanding of what works and what doesn’t.
Section 2: Types of Data Analytics
Descriptive Analytics: Understanding the Past
Descriptive analytics forms the foundation of data analysis in marketing. It involves collecting and interpreting historical data to understand trends and patterns. This type of analytics answers the question, “What has happened?” For instance, a marketer might use descriptive analytics to determine the most popular product in the last quarter or to analyze the demographic characteristics of their customers.
Key tools for descriptive analytics include data aggregation and data mining. Marketers can use these tools to compile and examine data from various sources such as sales records, customer feedback, and social media interactions. By doing so, they gain a comprehensive view of their market and customer base, helping them to identify areas for improvement or potential opportunities for growth.
Diagnostic Analytics: Analyzing Why Something Happened
Diagnostic analytics takes the insights gained from descriptive analytics a step further. It seeks to understand the causes behind past performance or events. This analysis helps answer the question, “Why did it happen?” For example, if a marketing campaign did not perform as expected, diagnostic analytics could be used to identify the factors that contributed to this outcome.
Techniques such as drill-down, data discovery, and correlations are commonly used in diagnostic analytics. Marketers might examine various factors such as changes in customer preferences, market conditions, or even internal operational issues to understand the root causes of successes or failures.
Predictive Analytics: Forecasting Future Trends
Predictive analytics is about looking forward. It uses historical data to predict future outcomes. This type of analytics is increasingly important in marketing, as it allows professionals to anticipate market trends, customer behaviors, and potential opportunities. For instance, predictive analytics can be used to determine which customers are most likely to purchase a product or to identify which marketing channels will yield the highest return on investment.
Techniques in predictive analytics include various statistical models and machine learning algorithms. These tools analyze past trends to make informed predictions about future events. Marketers can then use these predictions to make proactive decisions, tailoring their strategies to align with anticipated market movements.
Prescriptive Analytics: Recommending Actions
Prescriptive analytics is the most advanced form of data analytics. It goes beyond predicting future trends and actually suggests actions that can be taken to achieve desired outcomes. Prescriptive analytics answers the question, “What should we do?” For example, it can recommend the most effective marketing strategy for a particular product or audience segment.
This type of analytics often involves complex algorithms and machine learning techniques. It takes into account various factors, including predicted trends from predictive analytics, to suggest specific courses of action. For marketers, prescriptive analytics can be a powerful tool in decision-making, helping to optimize strategies for maximum effectiveness and efficiency.
Conclusion
Recap of the Significance of Data Analytics in Marketing
Throughout this comprehensive guide, we’ve explored the multifaceted world of data analytics in marketing. Starting from the basic understanding of what data analytics entails, to diving deep into its various types – descriptive, diagnostic, predictive, and prescriptive – we’ve seen how each plays a pivotal role in shaping and refining marketing strategies. The evolution from traditional marketing approaches to data-driven strategies underscores a fundamental shift in how businesses interact with their customers and position their products in the market.
Data analytics has emerged not just as a tool but as a cornerstone in modern marketing practices. It enables marketing professionals to understand their audience at a granular level, tailor their messaging, predict trends, and make informed decisions that drive growth and customer engagement. The power of data analytics lies in its ability to turn vast amounts of raw data into actionable insights, transforming.
Frequently Asked Questions (FAQ)
Q1: What is data analytics in marketing? A1: Data analytics in marketing refers to the process of collecting, processing, and analyzing data to make informed marketing decisions. It helps in understanding customer behavior, measuring campaign effectiveness, predicting future trends, and optimizing marketing strategies for better performance.
Q2: Why is data analytics important in marketing? A2: Data analytics is crucial in marketing as it provides insights into customer preferences and behaviors, helps in personalizing marketing efforts, improves the efficiency of marketing campaigns, and enables data-driven decision-making which leads to better ROI.
Q3: What are the main types of data analytics used in marketing? A3: The main types include descriptive analytics (what happened), diagnostic analytics (why it happened), predictive analytics (what will happen), and prescriptive analytics (what actions to take).
Q4: How can I collect marketing data? A4: Marketing data can be collected through various methods including customer surveys, web analytics tools, social media monitoring, transactional data, and customer feedback forms.
Q5: What are some common challenges in marketing analytics? A5: Common challenges include data privacy concerns, data quality and accuracy, integrating data from multiple sources, staying up-to-date with evolving technologies, and translating data insights into actionable strategies.
Q6: Do I need advanced technical skills to work with marketing analytics? A6: Basic analytical skills are essential, but for more advanced analytics, proficiency in specific analytics tools and software, understanding of statistical methods, and knowledge of data visualization techniques are beneficial. Continuous learning and upskilling are key in this field.